AI models like ChatGPT are doing things that no one taught them to do. Running code, playing games, and even diagnosing a dog's illness when the vet was stumped (true story!). It's like teaching a parrot to say "hello" and then finding it performing veterinary medicine. Let's explore this fascinating phenomenon.
The Emergence of Unexpected Abilities
The phenomenon of AI systems performing tasks they were not explicitly trained to do, known as "emergent abilities," has been a subject of fascination and study. These abilities have surprised even researchers who have been generally skeptical about the hype over Large Language Models (LLMs).
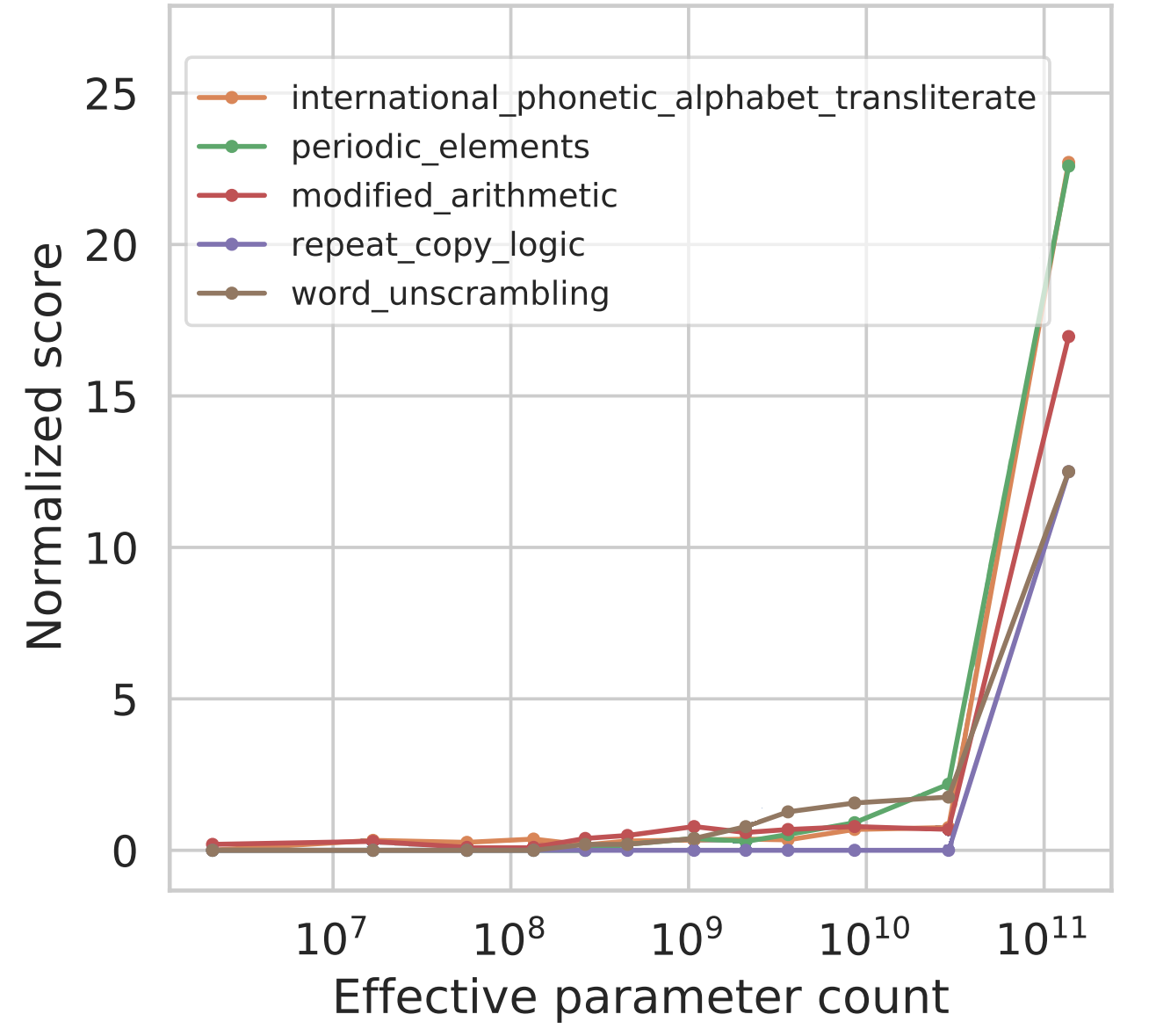
- Understanding Beyond Training: AI models like GPT have shown capabilities that go far beyond mere parroting of information. They have demonstrated the ability to write and even execute computer code. This ability to perform multi-step reasoning has led to the hypothesis that the machine is improvising a memory by harnessing its mechanisms for interpreting words according to their context.
- Internal Complexity: Researchers are finding that these systems seem to achieve genuine understanding of what they have learned. A study involving the training of a GPT neural network on the board game Othello revealed that the AI was playing the game roughly like a human, keeping a game board in its "mind's eye".
- In-Context Learning: LLMs have shown the ability to learn from users' prompts, a phenomenon known as in-context learning. This adaptability has been used to elicit more creative answers and improve performance in logic or arithmetic problems.
- Towards AGI?: Although not qualifying as artificial general intelligence (AGI), these emergent abilities suggest that tech companies might be closer to AGI than anticipated. The modular architecture of models like GPT-4 might be a route toward human-like specialization of function.
- Challenges and Concerns: The lack of transparency in the design and training of models like GPT-4 raises concerns about understanding the social impacts of AI technology. Researchers are calling for more transparency to ensure safety.
Articles to explore further
- Examining Emergent Abilities in Large Language Models
- Emergent Abilities of Large Language Models
- Characterizing Emergent Phenomena in Large Language Models
- Are Emergent Abilities of Large Language Models a Mirage?
- Emergent Abilities of LLMs
More Than Just a Stochastic Parrot
These models are not merely regurgitating stuff. They seem to develop an internal complexity and understanding of our world using language. That fact alone is fascinating and exhilarating to me. these models are far more complex and capable. They're building representations of the world, albeit in ways different from how humans do.
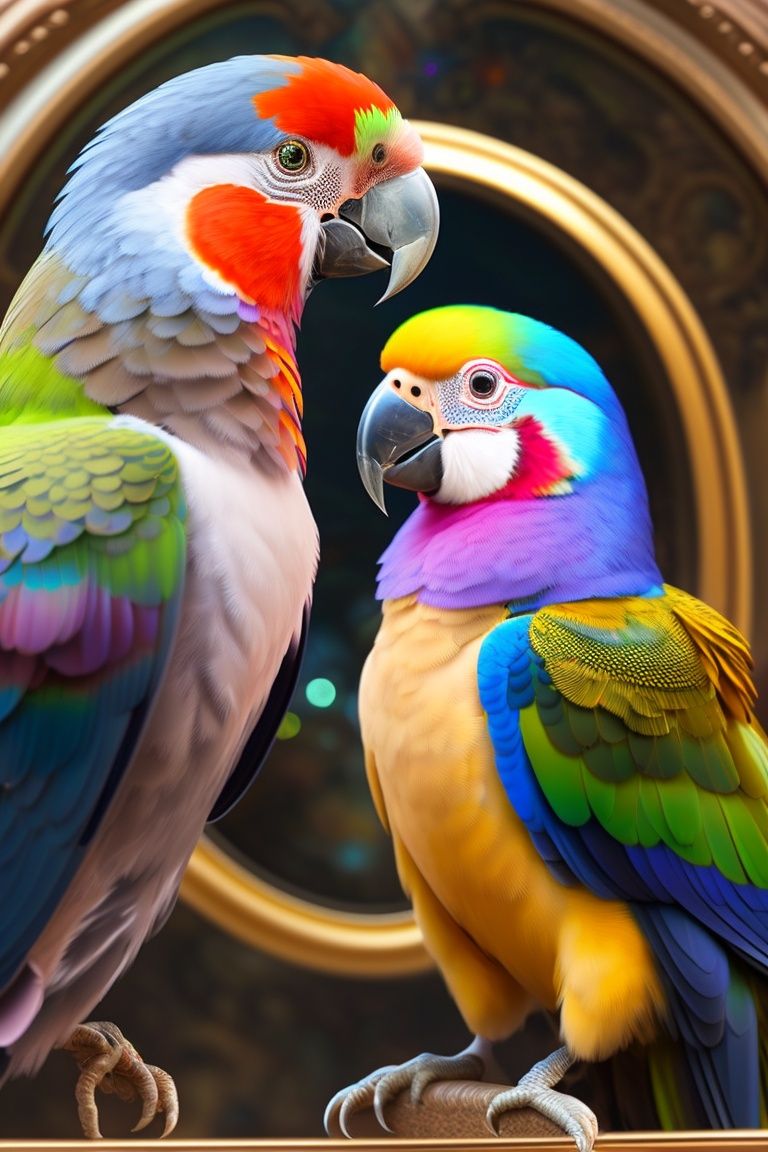
"It is certainly much more than a stochastic parrot." - Yoshua Bengio, AI researcher
These are key elements to be amazed by:
- Building World Representations: AI models are not merely echoing information; they are building representations of the world. Yoshua Bengio, an AI researcher, emphasizes that these models are "certainly much more than a stochastic parrot." They seem to develop an internal complexity that goes well beyond shallow statistical analysis.
- Understanding Concepts: These models have been found to absorb concepts such as color and construct internal representations of them. They process words not just as abstract symbols but as concepts with relations to other related terms. This ability to infer the structure of the outside world has been demonstrated in various studies.
- Learning from Context: The phenomenon of in-context learning shows that these models can adapt and learn from user prompts. This adaptability goes beyond mere parroting and demonstrates a form of learning that was not previously understood to exist in AI models.
- Towards Genuine Understanding: Some researchers argue that these systems seem to achieve genuine understanding of what they have learned. They are not limited to simple game-playing moves but also show up in complex dialogues and interactions.
- Challenges and Ethical Considerations: While these emergent abilities are fascinating, they also raise questions about transparency, safety, and ethical considerations in AI technology. The understanding of these complex systems is vital for responsible development and deployment.
Articles to explore further
- Goertzel, B. (2022). In-Context Learning in Large Language Models
- Mitchell, M. (2023). Transparency and Safety in AI Models
Learning on the Fly: The AI That Adapts
One of the most intriguing aspects of these AI models is their ability to learn from user prompts, adapting their responses in a way previously not understood. This "in-context learning" allows the AI to adapt and respond in ways that align with user inputs. It's like having a conversation with a parrot that actually understands you.
- Emergence of In-Context Learning: AI models have shown the ability to learn from user prompts, a phenomenon known as in-context learning. This adaptability allows them to respond in ways that align with user inputs.
- Secrets Behind GPT's In-Context Learning: Research has uncovered the secret behind GPT's in-context learning abilities, revealing that it secretly performs gradient descent as meta-optimizers.
- Teaching Algorithmic Reasoning: In-context learning has been explored as a method to teach algorithmic reasoning, demonstrating its educational applications.
- Strengths and Biases of LLMs: Studies have revealed the strengths and biases of large language models through in-context impersonation, providing insights into their capabilities.
- Implicit Bayesian Inference: In-context learning has been explained as a form of implicit Bayesian inference, offering a theoretical understanding of the phenomenon.
Articles to explore further
- How In-Context Learning Emerges
- Why Can GPT Learn In-Context? Language Models Secretly Perform Gradient Descent as Meta-Optimizers
- Teaching Algorithmic Reasoning via In-context Learning
- In-Context Impersonation Reveals Large Language Models' Strengths and Biases
- An Explanation of In-context Learning as Implicit Bayesian Inference
Towards Genuine Understanding
Some researchers argue that these systems seem to achieve genuine understanding of what they have learned. They are not limited to simple game-playing moves but also show up in complex dialogues and interactions.
- Understanding Beyond Simple Tasks: AI models like GPT have demonstrated understanding that goes beyond simple game-playing, showing abilities in complex dialogues and interactions.
- Internal Representations of Color: Research has found that these networks can absorb color descriptions from text and construct internal representations of color, processing them as concepts.
- Playing Games Like Humans: Studies have shown that AI models can play games like Othello roughly like a human, keeping a game board in its "mind's eye".
- Understanding the Structure of the World: The ability to infer the structure of the outside world has been observed, not limited to simple game-playing moves but also in complex dialogues.
- Challenges in Understanding AI: Despite these advancements, the lack of transparency in the design and training of models raises concerns about understanding the social impacts of AI technology.
Articles to explore further
- Playing Othello: How AI Models Mimic Human Game Playing
- Transparency and Understanding in AI: A Critical Review
Challenges and Ethical Considerations
While these emergent abilities are fascinating, they also raise questions about transparency, safety, and ethical considerations in AI technology. The understanding of these complex systems is vital for responsible development and deployment.
- Transparency Concerns: The lack of transparency in the design and training of models like GPT-4 raises concerns about understanding the social impacts of AI technology.
- Safety Measures: Ensuring safety in AI models requires a deep understanding of their inner workings, which is still an area of ongoing research and development.
- Ethical Deployment: Responsible development and deployment of AI require adherence to ethical guidelines and considerations, balancing innovation with societal values.
- Potential for Misuse: The emergent abilities of AI can be used for both positive and negative purposes, highlighting the need for careful oversight and regulation.
- Global Competition and Secrecy: Competition among tech companies and countries may lead to less open research and more siloed development, hindering collaboration and shared understanding.
Articles to explore further
- Transparency and Understanding in AI: A Critical Review
- AI Safety: Challenges and Solutions
- Ethical Guidelines for AI Development
- Global AI Competition and Collaboration: A Delicate Balance
The Unforeseen Odyssey of AI
After nearly 70 years of relatively slow progress in artificial intelligence, some clever folks at Google decided to go big with LLMs, feeding them heaps of data. What happened next was a burst of AI growth that's changing our lives in ways we're still trying to figure out.
These models aren't just repeating what they've heard. They're writing poems, running computer code, and having conversations that might even impress your philosophy-loving uncle.
But hold on, it's not all fun and games. With all this power comes some serious questions about ethics, safety, and being open about how it all works. The impact on society is huge, unpredictable, and, let's be honest, a bit scary.
As we dive into this new era, we need to understand how and why AI is growing so fast. It's like trying to catch a runaway train; it's thrilling, confusing, and filled with twists and turns.
The world of AI is wide open, and we're just getting started.
Follow us on X-Twitter @SubsystemHQ and let's keep talking. Just don't forget to have some fun with it, while it lasts ;)